✍️Research Review: Solving musculoskeletal biomechanics with machine learning.
- Martian to be
- Jul 21, 2023
- 2 min read
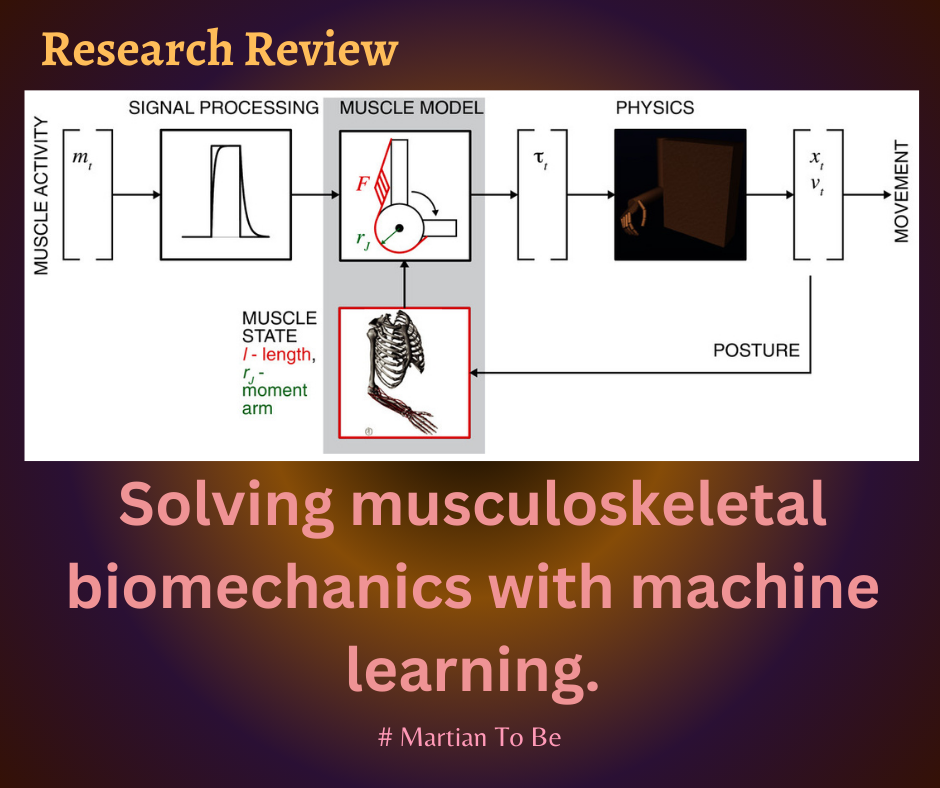
🌐Link to the article: https://peerj.com/articles/cs-663/
The paper explores the use of machine learning algorithms to approximate the posture-dependent moment arm and muscle length relationships of the human arm and hand muscles. The developed models demonstrate the future applicability of machine learning for musculoskeletal transformations in a variety of applications, such as in advanced powered prosthetics.
The introduction of the paper discusses the use of machine learning with artificial neural networks (ANN) in various applications, including driving vehicles, classifying media, and radiological diagnostics. The paper focuses on the potential of ANN to explore sensorimotor mechanisms and the high-dimensional computations involved in segmented body control. The complexity of this system offers unique challenges and opportunities for machine learning, particularly in the field of musculoskeletal biomechanics.
The paper does not provide a separate section for literature survey. However, it does mention previous studies that have used machine learning for musculoskeletal biomechanics, including the use of deep learning for the description of musculoskeletal dynamics and the use of polynomial structures to approximate posture-dependent muscle parameters. The paper also references previous studies that have used ANN for various applications, such as image classification and speech recognition.
The paper uses two machine learning models, light gradient boosting machine (LGB) and fully connected artificial neural network (ANN), to solve the problem of approximating the posture-dependent moment arm and muscle length relationships of the human arm and hand muscles. The input-output training and testing datasets, where joint angles were the input and the muscle length and moment arms were the output, were generated by a previous phenomenological model based on the autogenerated polynomial structures. Both models achieved a similar level of errors, with the ANN model requiring 10^6 samples and the LGB model requiring only 10^3 samples. However, the LGB models were about 39 times slower than the ANN models in the evaluation. The paper also discusses the workflow used for training and testing the models and the validation process used to prevent overfitting.
The paper uses input-output training and testing datasets, where joint angles were the input and the muscle length and moment arms were the output. These datasets were generated by a previous phenomenological model based on the autogenerated polynomial structures. The paper does not provide any other information about the data used.
The paper details the training outcome and the training dynamics for learning the transformation from joint posture to muscle lengths and moment arms. Both machine learning models, LGB and ANN, achieved a similar level of errors, with the ANN model requiring 10^6 samples and the LGB model requiring only 10^3 samples. However, the LGB models were about 39 times slower than the ANN models in the evaluation. The sufficient performance of developed models demonstrates the future applicability of machine learning for musculoskeletal transformations in a variety of applications, such as in advanced powered prosthetics.
The paper concludes that machine learning approaches, such as LGB and ANN, can be used to solve the problem of estimating muscle moment arms and their muscle lengths from joint angles. The developed models demonstrated sufficient performance and future applicability for musculoskeletal transformations in a variety of applications, such as in advanced powered prosthetics. The results of this study develop the potential for mechanistic machine learning approaches that utilize the musculoskeletal transformation for online control problems.
We value your ideas and feel free to comment below.💜
Commentaires